Acoustics Research Centre
Acoustics research has been conducted at Salford University for over 60 years. It is funded by research councils, national and international government bodies, and industry. Our research has fed into products that companies make and sell worldwide, as well as regulations and standards used in the UK, Europe and internationally.
‘outstanding impact demonstrated … live sports audio’
Engineering Panel, Research Excellence Framework (REF) 2021 feedback
We currently have about twenty research active staff and a lively community of PhD students. Staff and students have won numerous awards for their research.
We have world-class acoustics laboratories: listening rooms, reverberation rooms, anechoic chambers, an accredited calibration laboratory and state of the art equipment and instrumentation. Through the laboratories we are able to bring much of our fundamental research into real life applications.
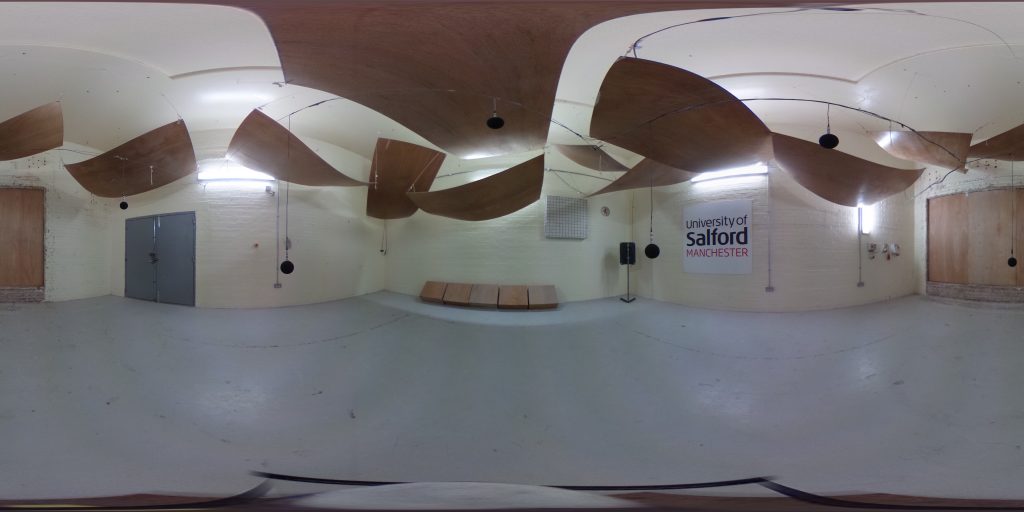
Industrial collaboration
We carry out a lot of testing and R&D work for companies, see Commerical R&D and Acoustic Testing.
Our research focus
- Acoustic measurements
- Architectural and Building Acoustics
- Audio Engineering
- Computer modeling of sound
- Environmental noise and soundscapes
- Materials for acoustics
- Psychoacoustics
- Public engagement
- Vibro-acoustics
Research Team
Acoustics research centre staff
PhD Opportunities
LAURA: The Leverhulme Trust Aural Diversity Doctoral Research Hub provides funded inter-disciplinary PhD and Masters training in the study of hearing and listening differences. First cohort starts 2024.
Contacts
Enquiries for specific areas of Acoustics research
Courses
Since the 1970s we have been teaching courses on Acoustics and Audio:
- MSc Acoustics
- MSc Audio Production
- BEng Acoustical and Audio Engineering
- MEng Acoustical and Audio Engineering
- BSc Sound Engineering Production
- Masters by research and PhD programme
Standalone Postgraduate Acoustics
In addition to the complete MSc, it is now also possible to take individual modules:
- Computer Simulation for Acoustics
- Environmental Noise Measurement and Modelling
- Immersive Sound Reproduction; Loudspeakers and Microphones
- Measurement, Analysis and Assessment
- Digital Signal Processing and Machine Learning
- Noise and Vibration Control
- Principles of Acoustics and Vibration
- Psychoacoustics and Musical Acoustics
- Room Acoustics